Welcome to CogSci Unpacked, an exciting new blog series dedicated to summarizing academic papers from the Cognitive Science, a CSS Journal. Our goal is to bridge the gap between academia and the broader public, fostering a better understanding of cognitive science and making it accessible and relatable to all. If you’re curious to dive even deeper, we invite you to explore the full academic paper.
Imagine you’re waiting for a train with your friend. While they’re in the bathroom, you get the tickets out of their suitcase and move them to your backpack for easy access. Your friend gets back and wants to double check the tickets. Where do you think they’re going to look for them?
If you guessed that they’ll look in the suitcase, congratulations: you just passed the False Belief Task! The task tests whether you can keep track of someone else’s beliefs, even when they differ from your own. This ability — often referred to as mindreading or theory of mind — is crucial for navigating social situations. It typically develops in humans before age 5, but there’s debate about whether it develops in other animals at all. This has sparked a “nature vs nurture” debate about where our mindreading abilities come from. Did we evolve dedicated cognitive mechanisms to perform social reasoning? Or do we learn this ability during our lifetimes through social interaction? Or from language?
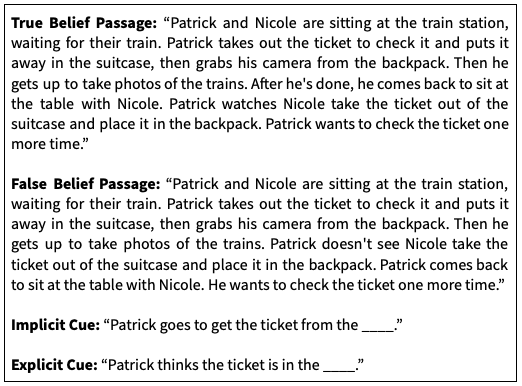
Figure 1. Example stimuli from the experiment. Patrick either sees the ticket being moved to the new location (True Belief) or is not aware that it has been moved (False Belief). Participants completed one of the two prompt sentences, which indicated Patrick’s belief either implicitly or explicitly.
In Trott et al. (2023), we tested if it was possible in principle to pass the False Belief Task based on language experience alone. We administered a version of the task to a Large Language Model (LLM): a neural network that learns to predict the next word in a sequence based on statistical patterns in language. LLMs serve as ideal guinea pigs for this question, because they don’t have any built-in knowledge or reasoning capabilities and no body: everything they learn comes entirely from exposure to language. If LLMs can correctly answer False Belief Task questions, it suggests that this behavior can be learned entirely from language input and doesn’t require any specialized cognitive equipment or physical experience in the world.
We presented GPT-3 (a LLM) with scenarios like the one above, followed by a sentence that indicated a character’s belief about an object’s location (e.g. “your friend thinks the tickets are in the ____”). We got GPT-3 to predict the final word in the sentence, and measured the probability that it assigned to the object’s start location (suitcase) versus the end location (backpack). In one condition (True Belief), the character saw the object being moved (so they should know that it is in the new location). In the False Belief condition, they didn’t see the object being moved (so they should still think the object is in the old location). We generated entirely new passages to ensure GPT-3 had never seen them before, and asked human participants to complete the same passages so we could compare their responses.
GPT-3 consistently assigned a higher probability to the start location in the False Belief condition compared to the True Belief condition (see Figure 2). For example, the model rated it as more likely that your friend would look for the tickets in the suitcase if they hadn’t seen you move them to the backpack. This behavior is consistent with the implied mental states of the characters. It suggests that the model is sensitive to information in the passage about what each character is likely to know, and uses this information to make predictions for later sentences describing their beliefs.
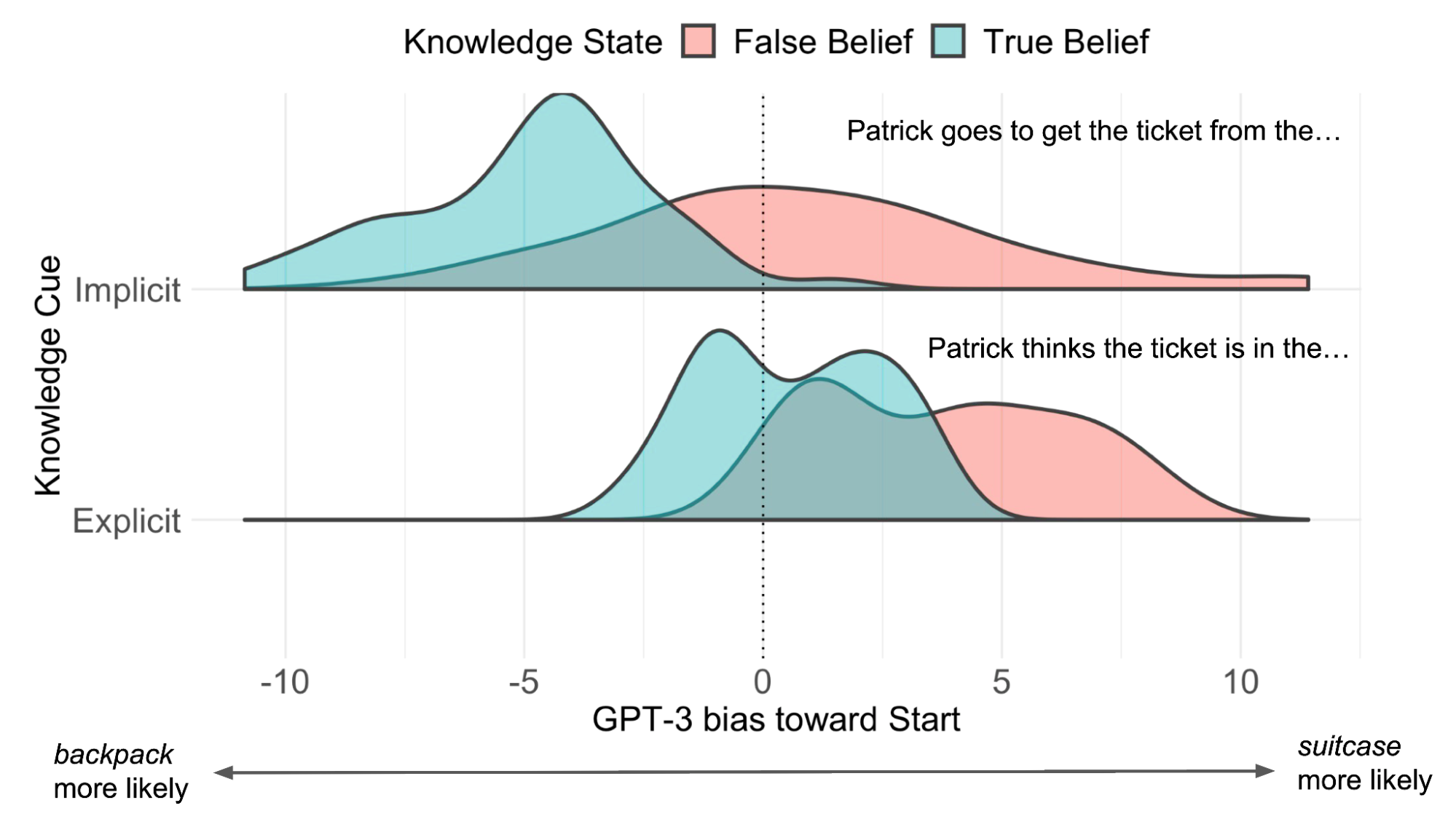
Figure 2. GPT-3 bias toward the start location compared to the end location: positive values indicate that p(start) > p(end). GPT-3 assigned higher probability to the start location on False Belief trials, indicated by the rightward shift of the red distributions. This was true both when the final sentence described the character’s belief implicitly (“Patrick goes to get the ticket from the ____”) or explicitly (“Patrick thinks the tickets are in the ___”).
Could humans be using the same kind of statistical linguistic information to perform the task? To test this stronger claim, we measured how much of the human responses could be explained by the LLM’s behavior. We found a significant in humans even after controlling for GPT-3’s performance, suggesting that humans are using information that isn’t captured by the model (see Figure 3). This was also reflected by a gap in performance at the task: while GPT-3 got 75% of the items right, human accuracy was 83%.
Do the results suggest that GPT-3 is actually representing beliefs?
The False Belief Task is often viewed as strong evidence of this ability in children and non-human animals. If we treat the test as equally informative in the case of LLMs, it indicates that GPT-3 has some ability to represent beliefs, albeit not as robustly as humans. Alternatively, you might argue that we know a priori that LLMs don’t represent beliefs: they’re just predicting the next word in a sentence after all! If so, the results indicate a problem with the False Belief Task: it can be passed by an agent that lacks the very ability it’s supposed to assess. One way to resolve this dilemma would be to look beyond the False Belief Task alone, and include other measures of belief representation — if GPT-3 consistently displays evidence of representing beliefs across a diverse range of tasks, it ought to increase our confidence that this result is not just a fluke. Thus far, ongoing work in this vein (including slight modifications to similar studies, and broader Theory of Mind benchmarks) has yielded mixed results.
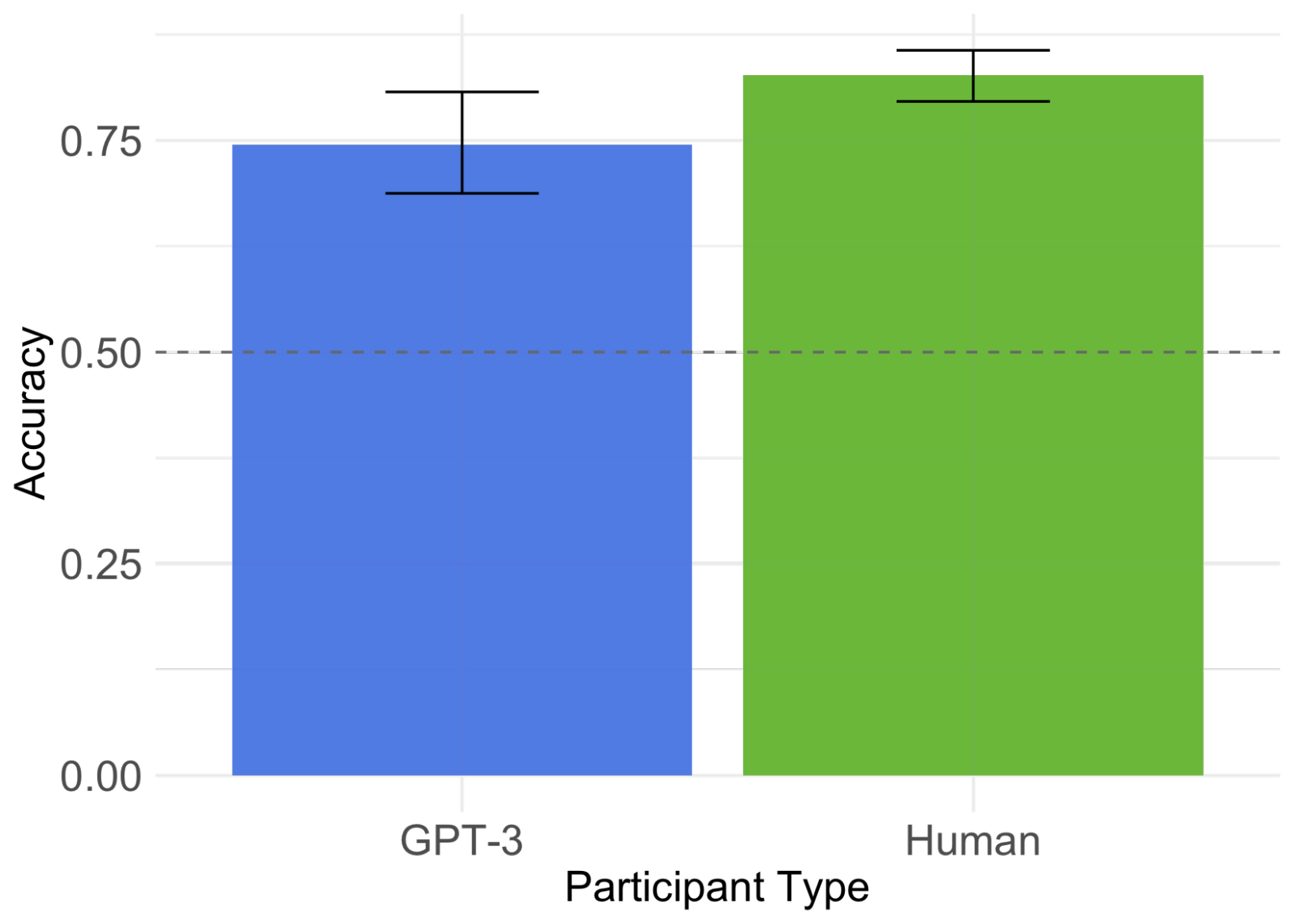
Figure 3. While GPT-3 was correct 75% of the time, human accuracy was 83%, and there was a marginal effect of knowledge state (True vs. False Belief) in humans that could not be accounted for by GPT-3 predictions.
Crucially, however, our results suggest that language statistics alone — at least insofar as they are learned by GPT-3 — are not sufficient to fully explain human belief sensitivity. Learning to understand others’ minds, therefore, may rely on linking linguistic input to innate capacities or to other embodied or social experiences.
_____